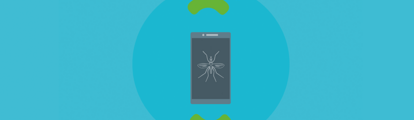
Hotline to Predictive Healthcare
What started as a phone line for people worried about dengue has grown into a sophisticated early warning system for outbreaks.
Epidemics are tracked in many different ways around the world. The CDC in the US uses interconnected hospital information systems to compare and integrate signals. Google Flu Trends tracks search queries for certain symptoms across time periods and locations. But in many regions high-tech methods like these aren’t possible – the right infrastructure isn’t in place, and it would be too difficult and expensive to implement. In Punjab, Pakistan, not all hospitals have a shared database of records, and the vast majority of the population doesn’t have Internet access. In these circumstances, how can we get the data we need?
Phoning it in
In 2011, there was an outbreak of dengue fever in Punjab that quickly escalated into the largest epidemic the region had ever seen. Dengue causes flu-like symptoms, rash and fever, and its severe form can be fatal, particularly for young children. “There was a sense of public panic, and authorities were unprepared for the scale of the outbreak, leading to massive queues at hospitals,” says Lakshminarayanan Subramanian, a Professor in the computer science department at NYU.
To calm the chaos, the government asked the pioneering Punjab IT Board (PITB) to launch a telephone hotline. The hotline was initially built for people to get a quick assessment of their symptoms, determine whether they needed to see a doctor urgently, and prevent hospitals from becoming overloaded. However, it also allowed the PITB to collect data about the number of suspected dengue cases in different areas, as well as keeping track of confirmed dengue cases from the referred hospitals.
Subramanian is a longstanding friend and collaborator of PITB Chairman Umar Saif, and the two decided to launch a research collaboration to explore the hotline’s potential for surveillance and forecasting. On analyzing the data, there was a clear trend between calling patterns and later outbreaks of dengue, with the hotline able to predict outbreaks two or three weeks before local hospitals could confirm (1).
The dengue forecast
“The call volume was substantial enough to create good forecasts – but the hotline itself was only the beginning,” says Subramanian. The team has now built up a whole ecosystem around the hotline, to track and predict dengue outbreaks. The system comprises more than 25 departments spanning 36 districts of Punjab, a plethora of standard containment practices, dashboards to share information with hospitals, and even public health teams who are sent out to perform containment activities, such as mosquito control.
“Tracking unconfirmed and confirmed cases of dengue by locality gave us very strong signals,” explains Subramanian. “Naturally, no single data source can be 100 percent relied on, and the hotline data can be skewed by people calling in about other diseases, reluctance to seek treatment after referral, and hundreds of other factors. To limit noise in the data we collect, we cross-reference it with data from other sources, such as hospital records.”
The hotline now also serves functions beyond its initial remit of advice on symptoms and hospital referrals. People can report environmental conditions that encourage mosquitoes (such as stagnant water), request mosquito fumigation of their neighborhood, and make complaints. “This adds value to the hotline and incentivizes its use,” says Subramanian.
About PITB
The Punjab Information Technology Board (PITB) was created in 1999 by the government of Punjab to help the region harness rapid advances in the field of IT, and build an internationally competitive IT industry. Today, PITB is working on over 60 projects and services ranging from utility billing, to e-stamping, to creating livestock databases. Since 2011, the Board has been led by computer scientist and entrepreneur Umar Saif, who is widely credited as a driving force behind the Pakistani government’s use of technology.
Punjab’s Dengue Activity Tracking System
As well as telephone and hospital reporting, the scheme makes use of smartphones to collect real-time data on cases, mosquito breeding grounds and prevention efforts.
- More than 25 departments across 36 districts of Punjab
- 1900 GPS-enabled smartphones used to log dengue cases and mosquito breeding areas
- 39,688 hotspots in four major districts (Lahore, Rawalpindi, Sheikhupura and Faisalabad) under weekly surveillance
- 145 hospitals with dengue data entry systems
- Over 6 million anti-dengue surveillance activities via android mobiles since launch
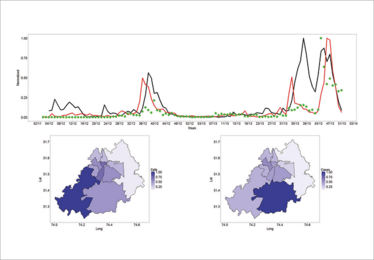
Figure 1. Trends in call volume and suspected dengue cases measured during 2012 and 2013. A) Time series of calls (red), suspected dengue cases (black), and awareness campaigns (green points). Scale normalized by dividing by individual maximum values. (B) Density map of calls across towns in Lahore. (C) Density map of cases across towns in Lahore. The legend is normalized by the maximum value. Lat, latitude; long, longitude. Reproduced from (1).
Subramanian affirms that while the ecosystem they developed started as an afterthought to the original hotline, a lot of follow-up work has gone into subsequent planning and validation, involving multiple collaborations with colleagues at NYU and in the UK. “Working with leading epidemiologists, we carried out detailed studies to maximize our effectiveness and ensure we are moving in the right direction,” he says.
The result is an early-warning system that is truly useful, despite its simplicity and low cost. “I think we have found a particular sweet spot of systems coming together to make our forecasting model work,” says Subramanian.
Spreading the word
Subramanian believes there are opportunities for similar hotline systems in other regions that lack widespread Internet access or linked hospital networks to take advantage of other forecasting tools; for example, Zika in Brazil, Ebola in West Africa, and even swine flu in certain areas.
The initial outlay is relatively modest, with most of the funds going on setting up and staffing the hotline and running public information campaigns. “The crucial first step is reaching a minimum call volume, because if you receive too few calls you won’t be able to build an accurate model,” says Subramanian. “Once you have the call volume, you need to get a good geographical spread and regularity of calls. If you can achieve sufficient scale and spread, you can begin to build on that initial momentum and create an effective forecasting tool.”
The strategy could also be applied in developed countries, where Subramanian envisages the hotline taking the form of an Internet-based hub that people can interact with – perhaps even with direct access to health professionals. There are many untapped opportunities within existing systems, too, says Subramanian. “Take the UK for example. The NHS already provides data with scale, spread, and regularity. Alone, this data might be too noisy to be useful, but if you start putting it together with health data that other organizations collect, you could have a very powerful predictive tool. And there are so many more examples like this around the world.”
Subramanian is convinced that there are massive opportunities to improve personal and societal healthcare using these data sources. “We just need to implement and utilize tools more effectively to improve disease prevention at very little cost.”
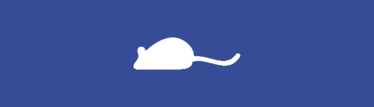
More Ways to Predict an Outbreak
From Mice to Men
New research shows that analyzing environmental change can help predict the risk of a zoonotic outbreak – when a disease leaps from animal to human. One such infection is Lassa fever, an acute viral hemorrhagic disease that usually infects West African rodents, but can spillover into human populations. A team at University College London looked at hundreds of past outbreaks of Lassa fever – taking into account location, land use, crop yields, weather conditions, and human population growth. The data from previous epidemics were combined with forecasts of climate change and population density to predict future prevalence (3). The team hope the model can be applied to other zoonotic diseases to help communities prepare for the future.
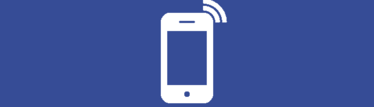
Mobiles and Mobility
Researchers at Harvard University are using mobile phone data to predict the spread of dengue (4). By looking at (anonymized) call records, the investigators were able to track the movement of people in Pakistan during a major dengue outbreak. Using the information gleaned from call records, alongside climate data, the researchers developed a novel transmission model, which can accurately forecast where and when the disease will strike next. This was the largest set of cell phone records ever analyzed to estimate mobility, spanning over 40 million users.
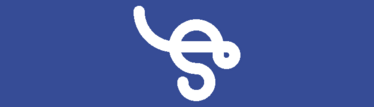
Catching Up to Ebola
The international community was slow to react to West Africa’s devastating 2014/15 Ebola epidemic. To help authorities act faster in future, epidemiologists at Columbia University conducted an analysis of data from the Sierra Leone Ministry of Health in the aftermath of the outbreak (5). They used a novel statistical model to give a detailed picture of the spread of Ebola through the country, and believe that real-time use of the model during future outbreaks could identify opportunities to curb transmission. In the midst of an outbreak, contact tracing can be too slow and cumbersome – the new model provides a faster way to track the spread of the disease, with minimal data required.
- NA Rehman et al., “Fine-grained dengue forecasting using telephone triage services”, Sci Adv, 2, e1501215 (2016). PMID: 27419226.
- Punjab Information Technology Board, “Dengue activity tracking system”, Available at: bit.ly/2frQ9k2. Accessed November 7, 2016.
- DW Redding et al., “Environmental-mechanistic modelling of the impact of global change on human zoonotic disease emergence: a case study of Lassa fever”, Methods Ecol Evol, 7, 646 (2016).
- A Wesolowski et al., “Impact of human mobility on the emergence of dengue epidemics in Pakistan”, Proc Natl Acad Sci USA, 112, 11887–92 (2015). PMID: 26351662.
- W Yang et al., “Transmission network of the 2014-2015 Ebola epidemic in Sierra Leone”, J R Soc Interface 12, 20150536 (2015). PMID: 26559683.
My fascination with science, gaming, and writing led to my studying biology at university, while simultaneously working as an online games journalist. After university, I travelled across Europe, working on a novel and developing a game, before finding my way to Texere. As Associate Editor, I’m evolving my loves of science and writing, while continuing to pursue my passion for gaming and creative writing in a personal capacity.