Cancer Static Shock
Are the metrics used to measure cancer drug efficacy inherently flawed?
When it comes to anticancer drug development, the difficulties of translating in vitro efficacy into clinical success are well known. But what if the metrics scientists use to measure a drug’s effect on cancer cell growth in vitro are inherently flawed?
A group of researchers from the Department of Cancer Biology at Vanderbilt University School of Medicine, US, believe that in vitro cell proliferation assays suffer from a number of biases (1). In response, they have developed a new metric, called “drug induced proliferation (DIP) rate”. Darren Tyson, Assistant Professor of Cancer Biology and lead author of the study, tells us more.
In what ways are current protocols flawed?
The use of a single measurement of cell number is widely employed across the scientific literature. Since it is based on a single time point measurement, we refer to this type of metric as “static”. Static metrics are flawed in multiple ways. Firstly, because cells grow exponentially, an untreated population will rapidly outgrow a drug-treated population. Perhaps more critically, the ratio of control to drug-treated cells will steadily increase over time, creating the illusion that a drug’s effectiveness is increasing over time. This is an example of what we call “time-dependent bias”. Another source of time-dependent bias in static metrics is that many drugs exhibit a lag time before their effect stabilizes within a cell population. This stabilization delay can cause drugs to appear more or less potent or effective than they actually are, which means ineffective compounds may be being improperly passed through the drug discovery pipeline or, conversely, effective drugs may be discontinued prematurely.
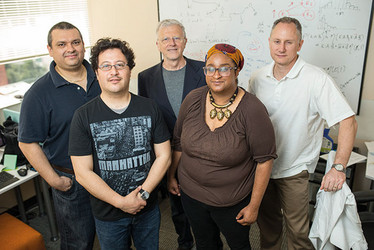
From left: Vanderbilt researchers Leonard Harris, Carlos Lopez, Vito Quaranta, Keisha Hardeman, and Darren Tyson (photo by John Russell).
How does your proposed DIP rate metric differ?
The DIP rate quantifies the growth of a cell population, or more precisely, the rate of change of a cell population size over time. Since the most important characteristic of a cancer drug is whether it can halt or reverse tumor growth, DIP rate is a natural and accurate metric: on a plot of cell population doublings (log2 cell counts) vs time, it appears as the slope of a line. As such, it is independent of time, once any delays in drug action have been accounted for. When developing the DIP rate metric, our biggest challenge was to determine when, after drug addition, a proliferation rate has stabilized. To support high-throughput drug screens, we had to develop reliable computational methods that could determine, in an automated fashion, when this occurs. The software is written in the R programming language and for academic applications can be obtained as free, open source software (2).
What are your next steps?
We want to measure DIP rates in large panels of cancer cell lines and search for novel molecular biomarkers of drug sensitivity, in addition to investigating DIP rate metric predictions of tumor cell responses in vivo. Both are translational tools for precision medicine.
We view the DIP rate as a metric of cell fitness in a particular environment, which extends beyond oncology. The DIP rate can act as a common currency, whether studying, for example, the influence of different drugs across a variety of cell lines, the effects of altering the microenvironment of stem cells, or the variation that exists at the single-cell level within a cell population (clonal heterogeneity, competition, or evolution).
- LA Harris et al., “An unbiased metric of antiproliferative drug effect in vitro”, Nat Methods (2016, Epub ahead of print). PMID: 27135974.
- D Tyson, LA Harris, “Code for Harris et al.,Nature Methods 2016.” Available at: bit.ly/23E8DOC
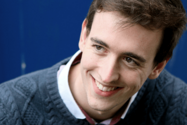
Over the course of my Biomedical Sciences degree it dawned on me that my goal of becoming a scientist didn’t quite mesh with my lack of affinity for lab work. Thinking on my decision to pursue biology rather than English at age 15 – despite an aptitude for the latter – I realized that science writing was a way to combine what I loved with what I was good at.
From there I set out to gather as much freelancing experience as I could, spending 2 years developing scientific content for International Innovation, before completing an MSc in Science Communication. After gaining invaluable experience in supporting the communications efforts of CERN and IN-PART, I joined Texere – where I am focused on producing consistently engaging, cutting-edge and innovative content for our specialist audiences around the world.