T Cell State of Mind
Comprehensive immune profiling may lead to better prediction of immunotherapy response
Jarret Glasscock | | 6 min read | Discussion
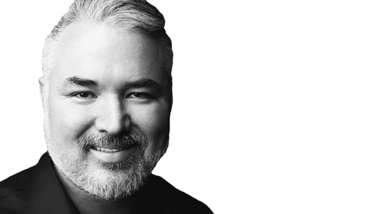
What must we do to improve outcomes for cancer patients? The answer is simple – gain a better understanding of the immune system. Accurate quantification of immune cells in the tumor microenvironment is key to understanding and treating the disease – and one of the most important (yet difficult) cells to quantify in the tumor microenvironment is the T cell. T cells have been shown to predict patient response to treatments such as anti-PD-1 therapy more accurately than on-label PD-L1 tests (1); therefore, to build a better biomarker, we must focus on characterizing these cells and examining their correlation with improved clinical outcomes.
The benefits of anti-PD-1 therapies are durable and robust, even in cancers with historically poor survival rates (2, 3). They work by modulating the immune system and have revolutionized the treatment of multiple cancer types, including melanoma and non-small cell lung cancer; however, despite their success in some patients, overall treatment response rate is typically less than 25 percent. Using immunohistochemistry (IHC) to measure PD-L1 levels and predict treatment response has historically been a popular choice, but a PD-L1-positive reading only modestly enriches for responders. Therefore, we still face a considerable gap in accurately identifying patients who will benefit from immunotherapies and sparing other patients the time, cost, and toxicity of unnecessary treatment.
Present predictions
Current efforts to improve patient response prediction focus on immune cell measurements and comprehensive immune profiling of the tumor. Immune profiling takes many forms – from IHC-based cell surface markers to multi-gene models – with each measurement method a step toward quantifying immune cell types in the tumor microenvironment. T cells are central players in predicting immunotherapy response – but the presence or absence of T cells in the tumor microenvironment is only one piece of the puzzle. T cell states (TCS) add another dimension altogether – specifically, the differentiation status of T cells that are naive, activated, effector memory, central memory, or exhausted. Exhausted T cells are particularly important for understanding the patient’s immune response, for even if a tumor is “hot” and contains a large number of T cells, they cannot fight cancer if they are exhausted (4).
A recent study demonstrated that nuanced TCS characterization is not only possible, but may also provide a more accurate basis for predicting anti-PD-1 response (5). The research represents a clear shift in diagnostics – from merely detecting the presence of T cells to detecting and quantifying cell states. Furthermore, specific functional roles are known to be associated with patient response; for example, in head and neck squamous cell carcinoma, higher levels of effector memory T cells are associated with treatment response, whereas exhausted T cells are associated with poor prognosis (6, 7). In non-small cell lung cancer, exhausted T cells are associated with response (8, 9). In fact, in many cancers, exhausted T cells are an important component in the anti-tumor immune response.
Estimating the presence of T cells in the tumor microenvironment begins with the creation of RNA models that uniquely identify cells using the most discriminatively expressed genes. A multi-gene immune cell modeling approach uses a two-step process: i) isolation or purification of specific immune cells and subsequent RNA sequencing to build a gene model for each cell type and ii) isolating RNA from future samples and using the purified models derived in step one as a “Rosetta stone” to report on the mixture composition of those samples.
Estimating the abundance of T cells based on the five cell states uses RNA models in a similar fashion. Instead of isolating cells by type and sequencing in the first step, T cells are forced into a particular state, then RNA sequenced. Data are then generated from differentially expressed genes that identify T cell subtypes and, finally, models capture the most important gene expression using machine learning. This data-driven approach creates an unbiased TCS biomarker with models that we hope are distinct enough to provide additional information in the context of tumor microenvironment immune profiling – ultimately helping to guide treatment decisions. As reported in both controlled in vitro and functional experiments (5), deconvolution of cell states from these models is not only possible, but has the potential to be used as an additional tool in predicting immunotherapy response.
The multi-gene RNA model approach to measuring T cell states is more advanced than current tools and does not rely on conventional single-analyte measurements. Traditionally, an easier (but less accurate) way to search for exhausted T cells was by measuring the protein PD-1; however, PD-1 is only correlated with, not specific to, exhausted T cells. The new, differentiated T cell models represent a more accurate method for predicting treatment response.
Next steps
Adopting advanced technologies is often difficult in the healthcare industry, but traditional methods of measuring TCS – particularly activated and exhausted subtypes – require functional assays or flow cytometry and are often incompatible with common clinical specimens. This modern method is easy to perform on commonly available clinical samples and even allows TCS characterization in formalin-fixed paraffin-embedded tissues – the most common format used today to store solid tumors following resection or biopsy.
The more we understand the immune composition and immune state in the tumor microenvironment, the closer we get to understanding which patients will benefit most from immunotherapies such as anti-PD-1 immune checkpoint inhibitors. No single analyte or approach has demonstrated such sensitivity or specificity to date; however, when we combine advanced tools such as high-throughput sequencing with machine learning to create complex models, we arrive at not only more accurate immune profiling, but also more accurate immune response prediction.
- AD Waldman et al., “A guide to cancer immunotherapy: from T cell basic science to clinical practice,” Nat Rev Immunol, 20, 651 (2020). PMID: 32433532.
- SC Wei et al., “Fundamental Mechanisms of Immune Checkpoint Blockade Therapy,” Cancer Discov, 8, 1069 (2018). PMID: 30115704.
- AB Warner et al., “Long-term outcomes and responses to retreatment in patients with melanoma treated with PD-1 blockade,” J Clin Oncol, 38, 1655 (2020). PMID: 32053428.
- S Sheth et al., “Durvalumab activity in previously treated patients who stopped durvalumab without disease progression,” J Immunother Cancer, 8, 650 (2020). PMID: 32847985.
- I Schillebeeckx et al., “T cell subtype profiling measures exhaustion and predicts anti-PD-1 response,” Sci Rep, 12, 1342 (2022). PMID: 35079117.
- GJ Hanna et al., “Frameshift events predict anti-PD-1/L1 response in head and neck cancer,” JCI Insight, 3, e98811 (2018) PMID: 29467336.
- MH Hong et al., “High CD3 and ICOS and low TIM-3 expression predict favourable survival in resected oesophageal squamous cell carcinoma,” Sci Rep, 9, 20197 (2019) PMID: 31882943.
- AO Kamphorst et al., “Proliferation of PD-1+ CD8 T cells in peripheral blood after PD-1-targeted therapy in lung cancer patients,” Proc Natl Acad Sci U S A, 114, 4993 (2017) PMID: 28446615.
- DS Thommen et al., “A transcriptionally and functionally distinct PD-1+ CD8+ T cell pool with predictive potential in non-small-cell lung cancer treated with PD-1 blockade,” Nat Med, 24, 994 (2018) PMID: 29892065.
Founder and CEO of Cofactor Genomics, San Francisco, California, USA.